Biometric Built-in ML AI Integrations: Unlock a World of Possibilities
Let’s dive deep into the exciting world of Biometric Built-in ML AI Integrations! Imagine a future where your devices truly understand you, anticipating your needs and adapting seamlessly to your unique patterns. This is no longer science fiction – it’s the reality of Biometric Built-in ML AI Integrations.
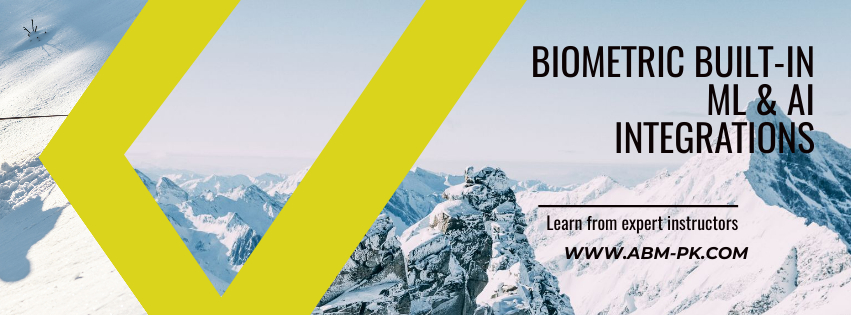
What exactly are Biometric Built-in ML & AI Integrations? In simple terms, it’s about seamlessly combining powerful AI and machine learning algorithms with your unique biometric data. This data could include your fingerprint, facial recognition, voice patterns, or even your unique typing rhythm.
Think of it this way: You’re unlocking your phone with your fingerprint. That’s a basic biometric. But with Biometric Built-in ML AI Integrations, your phone goes beyond simple unlocking. It learns your daily routines, anticipates your needs, and even adjusts settings based on your emotional state.
Here’s a real-life example: Imagine your smartwatch. With traditional biometrics, it tracks your steps and heart rate. But with Biometric Built-in ML AI Integrations, it goes further. It analyzes your sleep patterns, identifies stress levels based on your heart rate variability, and even adjusts your workout routine based on your performance history.
The possibilities are truly endless. Biometric Built-in ML AI Integrations can revolutionize how we interact with our devices.
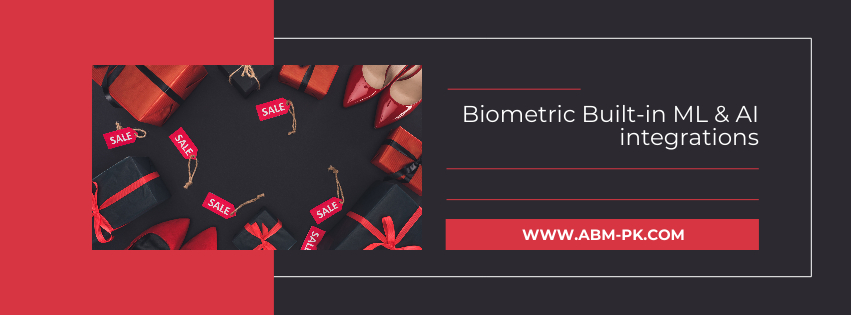
Imagine a world where:
Your smart home anticipates your arrival and adjusts lighting and temperature accordingly.
Your car automatically adjusts the driving mode based on your alertness levels.
Your workplace adjusts the ambient noise and lighting to optimize your focus and productivity.
But how does it all work? Biometric Built-in ML AI Integrations rely on sophisticated algorithms that continuously learn and adapt.1 These algorithms analyze your biometric data, identify patterns, and make intelligent predictions.2
For example: Your voice assistant learns your unique voice patterns, allowing for more accurate voice commands and personalized responses.3
The key to successful Biometric Built-in ML & AI Integrations lies in ensuring data privacy and security. Your biometric data is highly sensitive, so robust security measures are crucial.
Biometric Built-in ML AI integrations are revolutionizing the way we approach identity verification and security across various industries. These cutting-edge technologies are enhancing the accuracy, speed, and reliability of biometric systems, making them more robust and efficient than ever before. Let’s explore the top ways ML and AI are transforming biometric technology:
In recent years, there has been a significant advancement in the field of biometric technology, particularly with the integration of machine learning (ML) and artificial intelligence (AI) into biometric systems. This integration has led to the development of biometric built-in ML and AI integrations, which have revolutionized the way we authenticate individuals and secure sensitive information. In this essay, I will explore the various applications, benefits, challenges, and future prospects of biometric built-in ML and AI integrations.
One of the key applications of biometric built-in ML AI integrations is in the field of security and authentication. By combining biometric data with ML and AI algorithms, organizations can create highly secure and efficient authentication systems that are difficult to compromise. For example, facial recognition technology can be used in combination with ML algorithms to accurately verify a person’s identity, even in challenging conditions such as poor lighting or varying facial expressions.
Another important application of biometric built-in ML AI integrations is in the healthcare industry. ML and AI algorithms can be used to analyze biometric data from patients and detect patterns that may indicate potential health issues. For example, ML algorithms can analyze a person’s gait patterns and detect abnormalities that may indicate an increased risk of falling.
In addition to security and healthcare, biometric built-in ML AI integrations can also be used in a variety of other industries such as banking, retail, and transportation. For example, banks can use biometric authentication systems that are powered by ML and AI algorithms to verify customers’ identities and prevent fraud. Retailers can use facial recognition technology to personalize the shopping experience for customers, while transportation companies can use biometric data to enhance the security of passengers.
One of the key benefits of biometric built-in ML AI integrations is the increased accuracy and efficiency of authentication systems. By combining biometric data with ML and AI algorithms, organizations can create systems that are highly accurate and difficult to fool. This not only helps improve security but also enhances the user experience by reducing false positives and negatives.
Another benefit of biometric built-in ML and AI integrations is the ability to adapt and learn over time. By using ML algorithms, biometric systems can constantly improve their performance by learning from new data and experiences. This adaptability makes biometric systems more resilient to changes in the environment or attempts to bypass security measures.
However, despite the numerous benefits of biometric built-in ML AI integrations, there are also some challenges that need to be addressed. One of the main challenges is the potential for bias in the algorithms used in biometric systems. If the training data used to develop ML algorithms is biased, it can lead to inaccurate results and unfair treatment of certain groups of people.
Another challenge is the potential for privacy concerns. Biometric data is highly sensitive and can reveal a lot about an individual’s identity. Organizations need to be transparent about how they collect, store, and use biometric data to ensure that individuals’ privacy rights are protected.
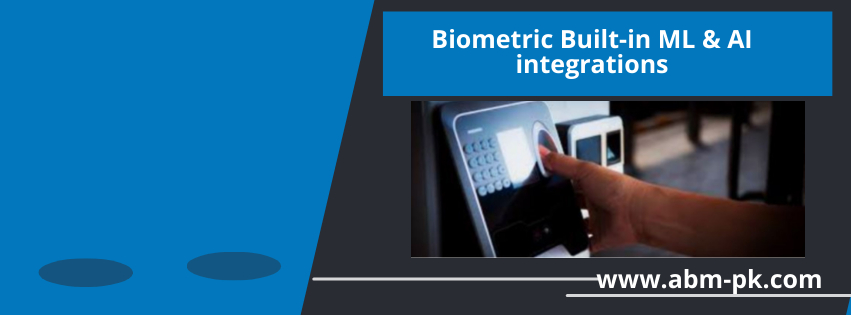
In the future, biometric built-in ML AI integrations are expected to continue to evolve and become even more sophisticated. As ML and AI technologies advance, biometric systems will become more accurate, efficient, and secure. We can expect to see more applications of biometric technology in various industries, as organizations seek to enhance security, improve user experience, and streamline operations.
Biometric built-in ML AI integrations have the potential to revolutionize the way we authenticate individuals and secure sensitive information. By combining biometric data with ML and AI algorithms, organizations can create highly secure and efficient authentication systems that are difficult to compromise. While there are challenges that need to be addressed, the benefits of biometric built-in ML and AI integrations are significant and will continue to drive innovation in this field.
1. Enhanced Facial Recognition
AI-powered facial recognition systems have become increasingly sophisticated, capable of analyzing facial features with remarkable precision. These systems can:
Rapidly process and authenticate biometric data for quick access in low-security environments like airports or government offices. Detect and prevent spoofing attempts by identifying unusual behavior or facial movements. Improve accuracy over time as more data is fed into the system
2. Advanced Behavioral Biometrics
Machine learning algorithms are now capable of analyzing unique behavioral patterns, adding an extra layer of security to traditional biometric systems. This includes:
Mouse activity and keystroke patterns, – Touch screen interactions, including press size, area, and pressure, Mobile device motion analysis
These behavioral biometrics can work silently in the background, potentially eliminating the need for annoying CAPTCHA challenges.
3. Multimodal Biometric Authentication
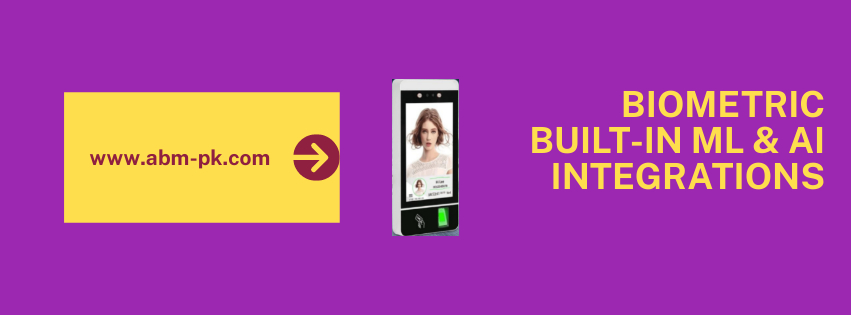
AI and ML enable the integration of multiple biometric modalities, significantly enhancing overall system accuracy and reliability. This approach:
Combines various biometric data points such as facial features, fingerprints, and behavioral patterns- Utilizes neural networks to process and analyze complex multimodal data- Improves security by making it more difficult for unauthorized users to bypass multiple authentication layers
4. Continuous Authentication
ML algorithms can provide ongoing authentication throughout a user session, addressing potential security risks. This feature:
Monitors behavioral patterns in real-time- Detects inconsistencies that may indicate an unauthorized user has gained access- Dynamically restricts access if suspicious activity is detected
5. Fraud Detection and Prevention
AI-powered biometric systems are becoming increasingly adept at identifying and preventing fraudulent activities. These systems can:
Analyze patterns of behavior to detect anomalies- Identify variations in biometric data that may indicate a threat- Provide real-time alerts to security personnel when potential fraud is detected
6. Adaptive Learning and Improvement
One of the most significant advantages of ML in biometric systems is their ability to learn and improve over time. This means:
Increased efficiency in distinguishing between close matches as more data is inputted
Continuous refinement of authentication algorithms, improved ability to handle diverse and challenging biometric data
7. Enhanced Data Processing and Analysis
AI and ML technologies enable biometric systems to process vast amounts of data quickly and accurately. This leads to: Faster authentication times in high-traffic areas
Improved ability to handle large-scale biometric databases, more efficient resource allocation in security operations
8. Integration with IoT Devices
Biometric Built-in ML & AI integrations are paving the way for seamless integration with Internet of Things (IoT) devices, expanding the reach and applicability of biometric security. This includes: Smart home security systems with facial recognition, Wearable devices that continuously authenticate users based on behavioral patterns, Vehicle access systems that use multimodal biometric authentication
9. Improved Accessibility and User Experience
AI-driven biometric systems are making authentication processes more user-friendly and accessible. This is achieved through: Touchless biometric solutions that are more hygienic and convenient, – Adaptive interfaces that cater to users with disabilities, faster and more intuitive authentication processes
10. Advanced Liveness Detection
ML algorithms are enhancing the ability of biometric systems to distinguish between live users and spoofing attempts. This includes: Detection of 3D facial structures to prevent photo-based attacks, Analysis of micro-expressions and involuntary movements, Integration of thermal imaging for additional verification
Now, let’s address some common FAQs:
Q: Is my data safe with Biometric Built-in ML & AI Integrations?
A: Yes, leading companies prioritize data security and privacy.
Q: Strong encryption and secure data storage are essential.
A: You should always choose devices and platforms that prioritize user privacy.
Q: How can I control my data?
A: Most devices and platforms offer granular control over data sharing and usage.
Q: You can typically adjust privacy settings to limit data collection and usage.
A: What are the potential benefits of Biometric Built-in ML & AI Integrations?
Increased convenience and efficiency in daily life. Enhanced security and personalized experiences. Improved health and well-being through proactive insights of access control Dubai.
In conclusion,
Biometric Built-in ML & AI Integrations represent a significant advancement in human-computer interaction. By seamlessly integrating your unique biometric data with powerful AI and machine learning, these technologies unlock a world of personalized and intuitive experiences.
While challenges related to data privacy and security remain, the potential benefits are immense. As the technology continues to evolve, we can expect to see even more innovative and impactful applications of Biometric Built-in ML & AI Integrations in the years to come. I hope this article provides a comprehensive overview of Biometric Built-in ML & AI Integrations. Please provide additional prompts if you’d like me to expand on specific aspects or address further questions.
Biometric Built-in ML & AI integrations are transforming the landscape of identity verification and security. As these technologies continue to evolve, we can expect even more innovative applications and improvements in biometric systems, leading to a safer and more secure digital world.